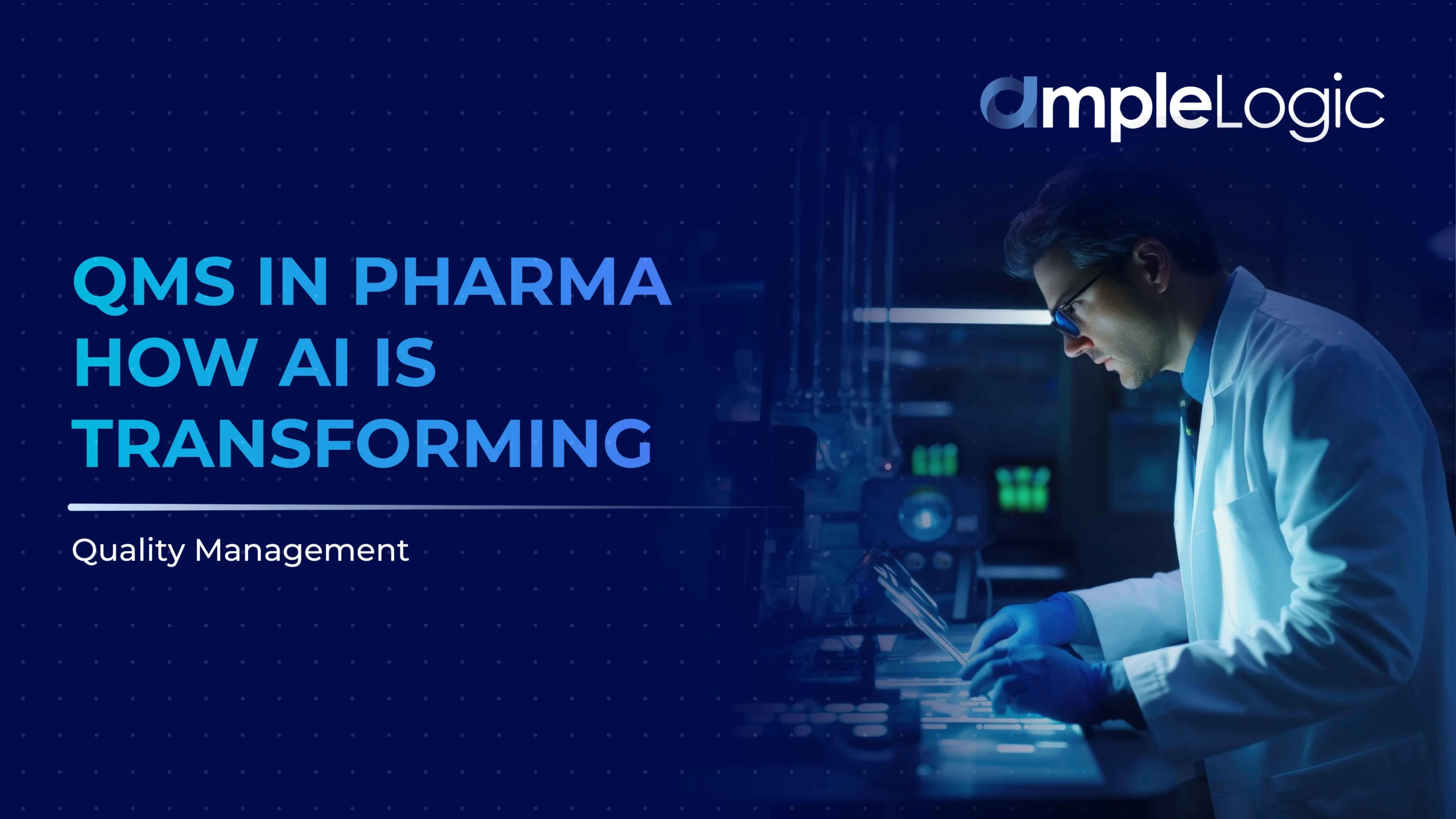
Artificial Intelligence has certainly become part of our day-to-day lives. It is latched to our day-to-day life processes and has made technology easy to use and super-interactive. As the pharmaceutical and Lifesciences industry indulges in various tasks on a day-to-day basis, there are several inefficiencies that AI can easily help mitigate. Maintaining superior quality in pharma and the lifesciences sector is essential. Â
In order to ensure continuous process and product quality, organizations use advanced Quality Management Systems. These solutions help streamline the process of identifying root causes of quality issues and render faster mitigation. However, by implementing AI in QMS in pharma, processes are further optimized to achieve maximum ROI. Let’s go deep down into understanding how AI in quality systems can level up your organization with faster quality management processes, ensuing up to 70% efficiency.Â
The Hidden Struggles of Conventional Pharma Quality Systems
Traditionally, EQMS software in pharma have various interconnected modules to solve quality-related issues. This can range from CAPA, Change Control, Out-Of-Specification (OOS) & Out-Of-Trend (OOT), Deviation Management, Vendor Qualification, Market Complaints Management, Audit Management, Incident Reporting, Product Recall & Quality Risk Management. Traditional quality management processes impact efficiency, compliance, and overall quality effectiveness. Â
- During CAPA Management, there are often struggles with delayed root cause analysis and ineffective tracking, leading to recurring issues. Change Control Management is often hindered by complex approval workflows, poor visibility, and compliance risks due to manual documentation. Â
- OOS & OOT Management suffers from delayed investigations and ineffective trend analysis, increasing compliance risks. Deviation Management lacks integration with CAPA and Change Control, causing fragmented data and inconsistent handling.Â
- Vendor Qualification Management is inefficient, with time-consuming assessments and poor performance tracking, leading to compliance risks. Market Complaints are often delayed due to inadequate tracking and poor integration with CAPA. Â
- Audit Management faces issues with manual tracking, limited visibility, and slow response times. Incident Reporting is plagued by inconsistent documentation and delays, affecting proactive decision-making. Â
- Product Recalls pose significant challenges due to slow batch identification and inefficient coordination, increasing compliance risks. Quality Risk Management lacks proactive identification, structured assessment methodologies, and integration with other quality control and quality assurance processes.
Overcoming these challenges requires modern, automated Pharma quality systems that streamline workflows, enhance visibility, and ensure compliance across all quality processes.
How AI is transforming QMS in Pharma
With the advent of new and emerging technologies in Life sciences, here is how AI can help you attain pharma quality assurance efficiently.
AI Application in Module-Based Quality Systems
1. Change Control Management
Streamline processes by automating tasks and reducing redundancy. It can assess risks and recommend change control measures based on historical data, saving time and effort. Quality Management Systems with AI capabilities also identify impacted departments and outline action plans, easing the workload for teams.
With sophisticated algorithms, QMS in pharma provides a comprehensive impact analysis, evaluating operational, equipment, and regulatory effects. Additionally, it helps define timeframes for completing change control, accelerating execution, and ensuring closure within specified timelines. This approach can improve cost-effectiveness by up to 70%.
2. CAPA (Corrective and Preventive Actions) Management
Detects quality trends and predicts potential issues before they escalate. It uses Root Cause Analysis (RCA) with AI-driven anomaly detection to identify systemic issues and suggests data-driven CAPA recommendations based on historical cases within Quality Management Systems. This leads to proactive problem resolution, faster root cause identification, and reduced quality incidents and non-conformances.
3. OOS (Out of Specification) & OOT (Out of Trend) Management
Applies predictive analytics to identify deviations in laboratory test results. Machine learning algorithms within QMS in pharma analyze historical data to predict potential OOS/OOT occurrences, reducing investigation time. AI-powered automation assists in root cause analysis, ensuring timely corrective actions and preventing future occurrences. The impact includes faster OOS/OOT resolution, improved regulatory compliance, and enhanced product quality control.
4. Deviation & Non-Conformance Management
Analyzes historical deviation patterns to predict process failures. Natural Language Processing (NLP) automates deviation categorization within quality management systems, reducing manual review time, while AI-based risk scoring prioritizes high-risk deviations for immediate resolution. The impact includes faster deviation investigations, minimized production delays, and data-driven risk mitigation strategies.
5. Vendor Qualification Management
Evaluates supplier performance based on historical quality metrics, audit results, and delivery timelines. Quality Management Systems with AI-driven risk assessment tools categorize vendors based on compliance history and product quality. Automated supplier ranking ensures that only reliable vendors are engaged, reducing supply chain risks. The impact includes enhanced supplier quality control, reduced risks of contaminated raw materials, and faster and more accurate material inspections.
6. Market Complaints Management
Utilizes sentiment analysis to monitor customer complaints for emerging quality issues. Machine learning in QMS in pharma predicts potential recalls by analyzing deviations, batch failures, and market complaints, while AI-powered automation streamlines complaint investigation and resolution processes. The impact includes proactive issue identification before recalls happen, improved response time for complaints, and reduced brand damage due to quality failures.
7. Audit & Inspection Management
Automates audit planning, scheduling, and documentation tracking. Machine learning within EQMS software analyzes previous audit reports to identify recurring compliance gaps, and AI-powered chatbots assist auditors in retrieving regulatory information instantly. This results in streamlined audit processes, faster compliance issue detection, and a reduced manual documentation burden.
8. Incident Reporting
Automates real-time incident logging and categorization. AI-powered risk assessment tools within EQMS software analyze incident severity and recommend corrective actions. Predictive analytics help in identifying potential hazards before they become critical issues. The benefits include faster incident resolution, improved workplace safety, and enhanced regulatory reporting compliance.
9. Product Recall Management
Enhances recall efficiency by predicting potential recall scenarios before they occur. Machine learning in EQMS software analyzes deviations, complaints, and quality trends to identify products at risk of recall. AI-powered automation streamlines recall execution, ensuring faster product retrieval and regulatory compliance. The impact includes improved response time, minimized financial loss, and reduced brand reputation damage.
10. Risk Management (ICH Q9 Compliance)
Applies predictive analytics to assess and prioritize risks in manufacturing. It uses Failure Mode and Effects Analysis (FMEA) to evaluate potential failure points, and AI-driven simulations test different risk mitigation strategies before implementation within EQMS software. The benefits include proactive risk prevention, improved decision-making with real-time risk insights, and reduced product recalls and compliance failures.
See more – Simplifying Deviation Management with AI
AmpleLogic’s AI integrated Quality Management System
AmpleLogic’s AI integrated EQMS software is designed to transform quality processes in the life sciences industry by integrating automation, AI-driven analytics, and regulatory compliance. Built on a Low-Code/No-Code platform, the software offers seamless customization without extensive coding. It streamlines deviations, CAPAs, change controls, audits, training management, and document control while ensuring compliance with FDA, MHRA, EU-GMP, and other global regulations.
Key features of AmpleLogic’s AI QMS:
- AI Change Control Recommendation System: Analyses historical data, regulatory guidelines, and risk factors to suggest optimized action plans, categorize change requests, predict potential impacts, and recommend approvals to reduce delays and compliance risks.Â
- AI in Deviation Management: Enhances root cause analysis by identifying patterns across multiple deviations. Machine learning algorithms predict deviation trends, recommend risk-based resolutions, and ensure timely closure of deviations.Â
By leveraging AmpleLogic’s AI-powered QMS, pharmaceutical and biotech companies can achieve operational excellence, accelerate approvals, and maintain high-quality standards efficiently at reduced costs. AI-integrated quality management system ensures proactive risk mitigation, streamlined workflows, and enhanced compliance, paving the way for a smarter and more efficient pharmaceutical industry.Â
For a free demo, you can head on to our website AmpleLogic, and get more information. Also, if you found this blog informative, find more such interesting articles here – AmpleLogic Resources!