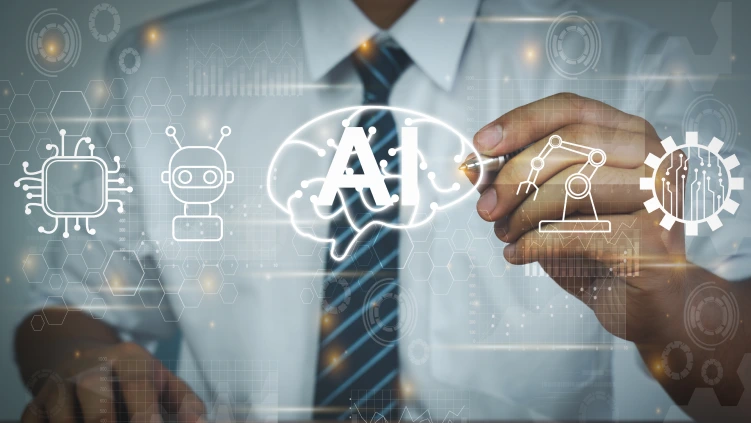
There is no doubt that we have entered the new industrial revolution, commonly referred to as Industry 4.0. Digitalization is transforming the way products are being manufactured, controlled, distributed and improved upon.
For years though, pharmaceutical companies were lagging behind due to the high cost of process shifting imposed by stringent GMP compliance requirements by US FDA and MHRA, lengthy software implementation timelines and high validation cost . Reasonably so, as caution is necessary before adopting any new technology in such a delicate field.
However, the pandemic has forced the entire Pharma industry to kick up a gear. Rapid increase in demand especially for biopharmaceutical products and medical devices over the past couple of years revealed inabilities of traditional setups to adapt to sudden changes in market requirements.
Today organizations are spending a good amount of money on process deviations. An average cost of deviation (start to end) will cost nearly USD 40000 to 200000 per deviation. There are certain steps in deviations that can be digitalized with the AI/ML technologies, resulting in reduced cost of deviations by 60%.
As a result, pharmaceutical industry has been propelled into Pharma 4.0, fueled by sophisticated technological solutions that significantly improve efficiency of an average manufacturing unit.
How can AI/ML be used in Quality Management Processes?
Quality Management Systems (QMS) stand as the bedrock of ensuring consistent product quality and impeccable service delivery in various industries. These systems streamline and automate an array of critical business processes, ranging from product design and Standard Operating Procedure (SOP) development to management reviews, audits, and corrective actions.Â
A typical pharmaceutical plant collects a remarkable amount of data as part of the quality assurance process. By having to document every part of the process to ensure quality tracking and error traceability, even a mid-sized manufacturing facility’s QMS software will collect enough data in one year to overwhelm an average server. Traditional means of storing data on local servers quickly become cumbersome, expensive and difficult to manage, as they require almost constant investments into equipment maintenance and capacity increases.
Storing all the information is only part of the problem, solvable by relying on cloud services. An even bigger issue lies in the inefficiency of such a practice. Mountains of data remain un-analysed, unused and practically forgotten. The only purpose becomes compliance, missing out on valuable opportunities to spot mistakes as they happen and gather valuable insight through in-depth analysis.
The amount of stored data only continues to grow with every new device and process being introduced into the system. Unless indexed and stored in such a way to allow effective analysis, both in real time and retroactively, it is essentially wasted.
However, the sheer volume and diversity of data and documents handled within QMS operations present formidable challenges. Manual processing of such extensive information not only introduces the risk of human error but also jeopardizes product integrity and consumer safety. Some valuable uses are mentioned below:
Complaints Management
As an example, procedures for handling customer complaints are defined as part of the Good Manufacturing Practice for the pharmaceutical industry. Since these usually relate to substandard medicines or medical devices, that need to be addresses immediately and through an effective course of action.
AI and ML technologies are increasingly employed to automate the intake, triage, and classification of complaints sourced from unstructured channels like emails and chats within Quality Management Systems (QMS). Machine intelligence is utilized to detect trends and identify recurring issues, streamlining the risk assessment process by automatically categorizing risks for prompt evaluation. Furthermore, resolved or ongoing complaints are analysed and aggregated to provide recommendations for potential product recalls or risk mitigation actions, all executed seamlessly through automated processes.
Market complaints can be minimized by applying the ML on the existing complaint data, analyzing the past root causes and applying them before a batch is released to the market. By choosing an AI-driven solution that meets all the necessary regulatory requirements, companies can avoid costly mistakes as well as public relation disasters due to a slow response to a problem.
CAPA Effectiveness
The traditional means of identifying, reporting and fixing problems are highly inefficient compared to possibilities offered by AI. 21 CFR (part 11) states that every pharmaceutical manufacturer is obliged to establish procedures to implement corrective and preventive action in case of errors (the CAPA system). In other words, to identify the underlying cause of the detected issue, resolve it and prevent it from happening again, as well as to avoid such problems in the first place.
The usual way of handling errors is to manually maintain the CAPA register, monitor and track the actions taken, all the while relying on the employees to remain vigilant in reviewing logs, ensuring consistency in following procedures and being as productive as possible to respect the requested deadlines.
By evaluating recurrent issue investigations and using prior root cause analysis data to suggest containment and remedial actions while reducing redundancy. AI-based solutions help Quality Management Systems accelerate root-cause analysis prioritising risks helps to avoid quality accidents in the future and guarantees data-driven confirmation of the effectiveness of corrective and preventive action.
Risk Mitigation
Risks related to complaints, non-compliance, inspections, training, and audit findings are identified by an AI-enabled QMS system. In addition to identifying risk patterns, AI technology suggests risk-mitigation strategies according to the nature of the danger. The system displays the following steps, such as mitigate, transfer, eliminate, and accept, for each risk that has been discovered.
Furthermore, based on the previous Out-of-trend (OOT) and Out-of-specification (OOS) results the software is able to predict the tentative values that can be raised for specific products before they get tested.
Modern technology aims to solve this problem and offer opportunity for continuous improvement. Big data, artificial intelligence (AI), machine learning (ML) and deep learning (DL) are tools that turn these mountains of unusable pieces of information into valuable pools of data, insights and predictions. Data is practically useless – knowledge is what is valuable. These tools do exactly that: turn data into knowledge.
Benefits of Artificial Intelligence and Machine Learning in Quality Management
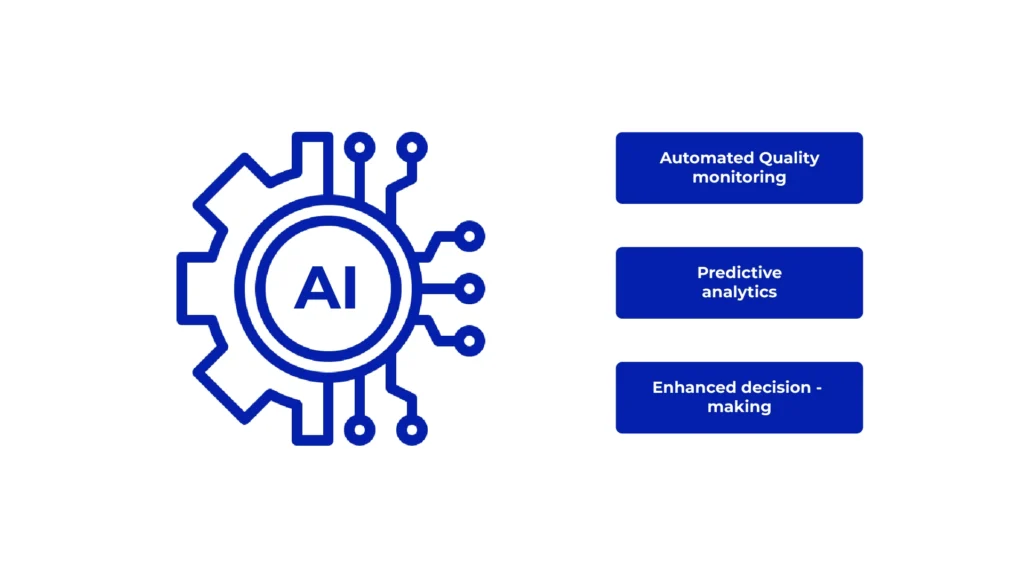
Automated Data Analysis: AI and ML algorithms can analyze vast datasets in real-time, enabling quick identification of patterns, anomalies, and trends. This automated analysis streamlines quality control processes and allows for proactive detection of issues before they escalate.
Predictive Maintenance: ML algorithms can predict equipment failures and maintenance needs based on historical performance data. By forecasting maintenance requirements, organizations can minimize downtime, reduce costs, and ensure consistent product quality.
Enhanced decision-making-The integration of AI and ML into Quality Management enables enhanced decision-making capabilities across various facets of operations. By leveraging advanced analytics and predictive models, organizations can make informed decisions regarding maintenance schedules, resource allocation, supplier selection, risk management strategies, and continuous improvement initiatives.
All these processes can be done quickly, even on an extremely large amount of data. As a result, these technologies produce meaningful insights that can solve problems, predict likely scenarios, or serve to improve the manufacturing processes.
This greatly simplifies the procedure of gathering data from different parts of the manufacturing process, from various devices and systems, even from separate departments, without having to create uniform reports or formatted data sheets. Raw data is being indexed, stored and then quickly analyzed whenever the need arises, thus significantly improving the data analytics in QMS.
Challenges with integrating Artificial Intelligence and Machine Learning into Quality Management
The organizations do not need to switch to an AI based QMS Software. Instead they can apply the AI/ML concepts on top of the existing QMS systems data. There are tested and proven practices that can ensure that the transition into the digitalized environment will be as seamless as possible, while reaping all the benefits that these sophisticated tools can offer.
Aside from lack of knowledge and capacity to handle such a switch on their own, most plants are faced with long and resource-consuming processes of new technology implementation. By choosing low code solutions, digitalization can be implemented quickly, efficiently and without the need for extensive changes in everyday procedures.
How can businesses ensure that they are getting the most out of AI and Machine Learning regarding Quality Management?
Plants should rely on SaaS (Software as a Service) providers that have experience and expertise in implementing AI into (bio)pharmaceutical setups. The supplier takes over the responsibility of implementing the technology and becomes a trusted partner that will guide the transition from beginning to end.
Future of Quality Management with the Integration of Artificial Intelligence and Machine Learning
Artificial intelligence is undoubtedly a valuable asset that will most likely remain on the forefront of digitalization, guiding the pharmaceutical industry into the 4.0 future. GMP standards implementation in compliance with US FDA, MHRA and other regulatory bodies, current data collection and processing makes pharmaceutical industry rigid, reactive instead of proactive, and slow.
Introducing new technologies into the existing practices will also introduce the ability to predict possibilities and resolve issues before they occur, improve processes continually with verification following alongside of it, and enabling more agile adaptability to the market.
Even though Pharma is one of the most controlled industries in the world, mistakes still happen, and recalls are necessary. By introducing AI and Machine Learning in Quality Management System, we can expect for quality control to be improved and brought into real time, to remove the need for recalls and actions further down the line, as the products leave the production facility. The future of quality assurance lies in AI and it’s coming to Pharma, one digitalized plant at the time.